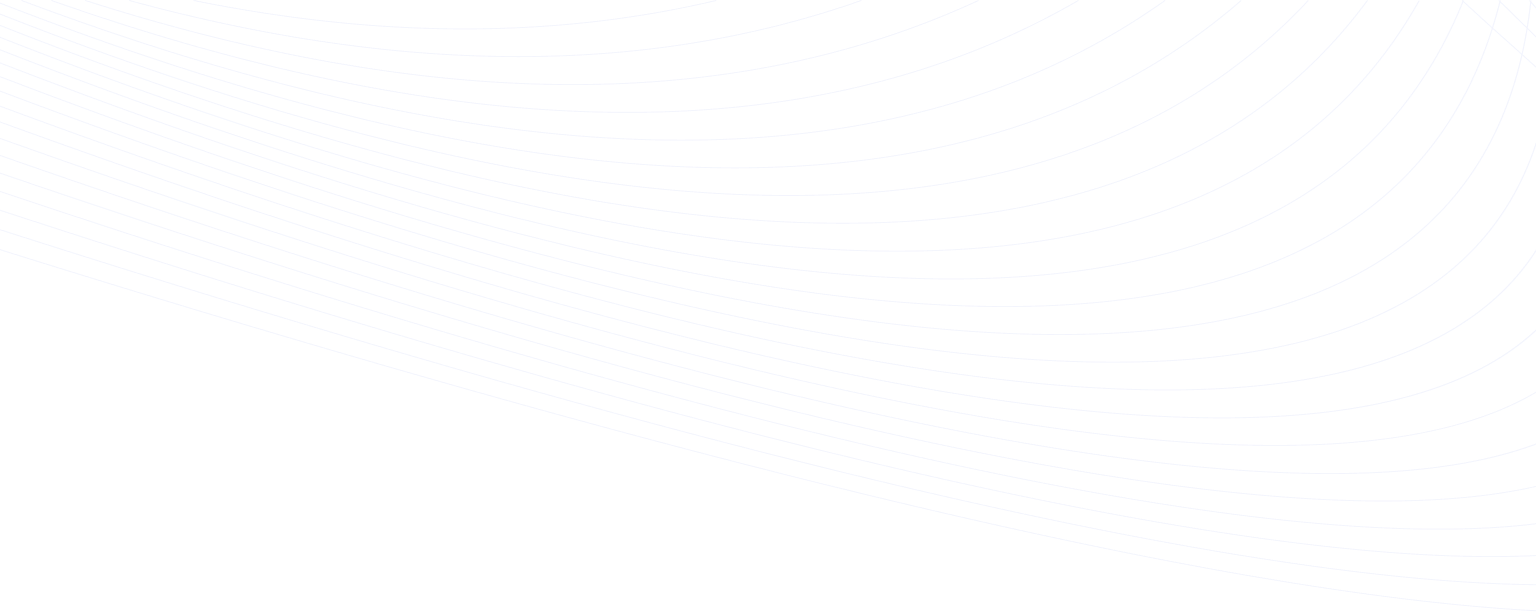
5 example use-cases for real-time data processing
The applications for real-time data processing are diverse and far-reaching. This article highlights just a few.
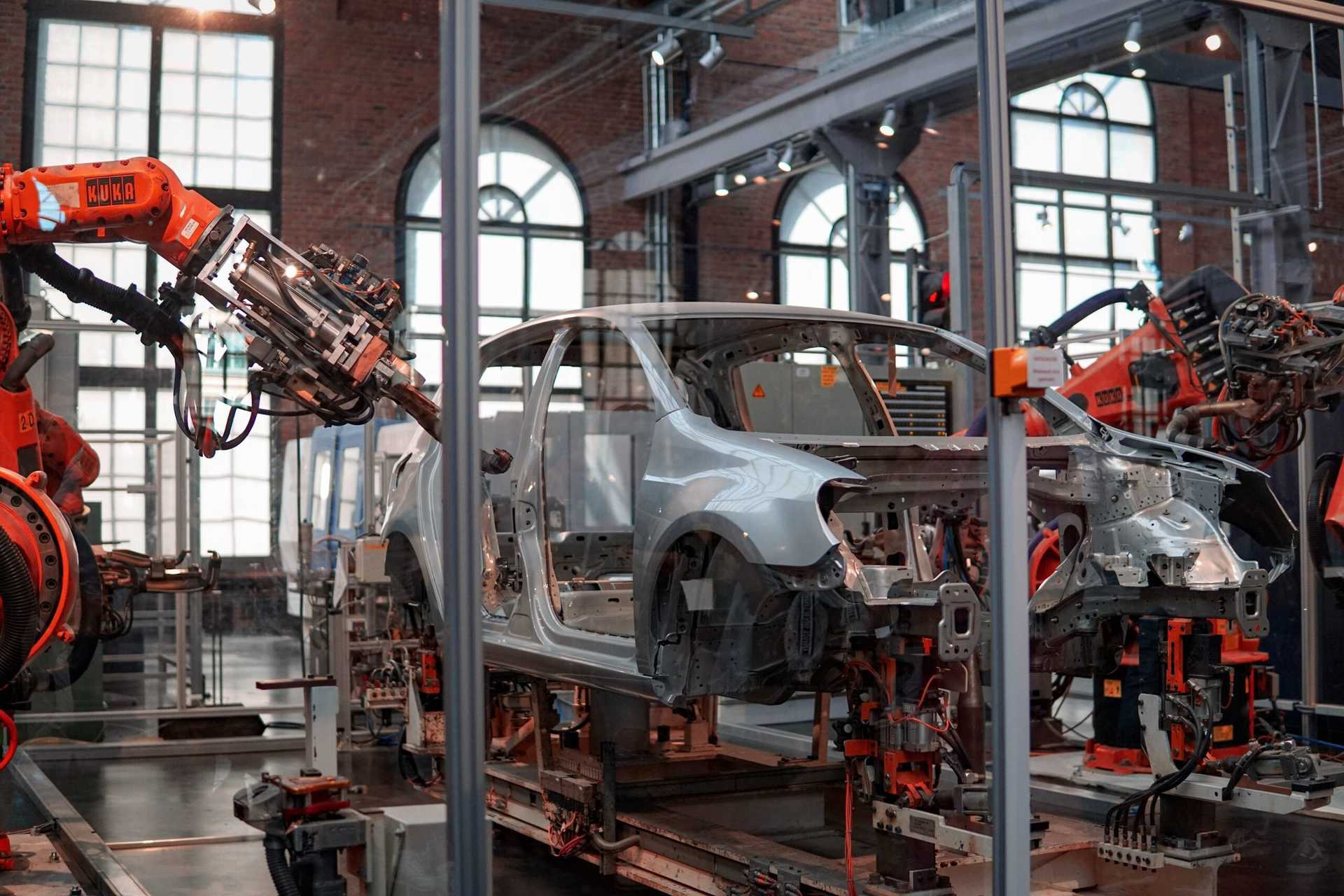
What is real-time data?
Real-time data is data that is collected, processed, stored, and put to work with millisecond latency.
The ability to stream data continuously has revolutionized a multitude of industries. This relatively new technology is gaining traction as it gets easier to implement.
Intuitively, it’s easy to understand the value of real-time for our data-driven world. But what does it look like in action? To answer this question, we’ll explore a few common real-time data use-cases.
Use-cases for real-time data processing
The applications for real-time data processing are diverse and far-reaching. This article highlights just a few.
Though these examples apply to different industries, they have some things in common. These shared themes illustrate the benefits of real-time data processing. They include:
- Granularity and personalization. From individualized marketing to daily sales plans, real-time processing allows you to break data down and use insights on a smaller scale.
- A backbone for other trending technologies. To use machine learning, artificial intelligence, and the Internet of things, you need a real-time data framework.
- Flexibility and timeliness. Getting insights instantly allows you to pivot quickly and stay ahead of the curve.
1. Manufacturing
The manufacturing industry is on the brink of a tech-driven renaissance*, thanks in large part to the Internet of Things (IoT) and real-time data analysis.
Since the industrial revolution, manufacturing has become increasingly streamlined, efficient, and automated. Though machines and robots are ideal for repetitive tasks, they’re not infallible. Monitoring is required to avoid performance issues and equipment downtime, which can cost companies millions.
IoT sensors can help by continuously monitoring each individual component. The data they collect can be analyzed in the cloud in real time, where potential issues can be identified early. This also reduces the need for frequent testing by human workers.
Real-time analytics in manufacturing is more than just a safety net against equipment failures. It also provides much more detailed insight into the manufacturing process. This allows manufacturers to make improvements, such as:
- Increased efficiency. Saving time, money, and resources across processes.
- Fine-tuning processes. Getting the most of each piece of equipment and each process.
- More flexible manufacturing. Adapting to fluctuations to stay efficient in all situations.
- Improved equipment longevity and availability. Using data to optimize maintenance is better for equipment.
- Higher product quality. Better maintained equipment and well-designed processes yield better products. Real-time analytics is also valuable for QA testing.
Industrial manufacturing has a huge physical component, which is costly to upgrade or replace. So, the shift to real-time insight in manufacturing is happening slowly. To see what the future might hold, we can look to one industry that is ahead of the curve. The automotive industry, which is known for adopting technological advancements pretty quickly, is already putting real-time analytics to work across the globe.
*Real-time is just one facet of a the technology changes on the horizon for the manufacturing industry. If you want to learn more, check out HiveMQ’s whitepaper on smart manufacturing.
2. Fraud detection
Think about the last time you swiped a credit or debit card. First, the card reader showed a “Processing Transaction” screen. Within a few seconds, your transaction was approved.
That small window of time is all that your financial institution had to decide whether your transaction was fraudulent, and stop it if necessary.
Because credit and debit card transactions involve near-instantaneous communication between the retailer and the financial institution, fraud analysis must be done in real time.
Real-time fraud analysis looks for specific patterns including:
- Grouping of transactions
- Unusually large transactions
- Unusual timings of transactions
- Small purchases followed by a huge purchase
- Transactions in locations that would be difficult or impossible to get to in a specific time.
The exact parameters of these red flags will differ across cases and evolve over time. Institutions use advanced AI and machine learning algorithms to adapt to new data and detect fraud more effectively. Real-time data is not only key to the effective use of these algorithms; it also powers them.
The applications of real-time analytics for fraud go beyond simply bouncing credit card transactions. They can help alert victims of an identity breach, and detect other types of fraudulent activity, like loan applications or insurance claim fraud.
3. Marketing and advertising
Good marketing requires a deep understanding of a business’s customer base. Only data can help do this well; fortunately, retailers have access to more data than ever before. This includes:
- Product-centric sales tracking. How is each product or offering selling by location or over time?
- Demographic information. Online retailers track variables such as the customer’s location, age, preferences, and income level. Loyalty programs allow data to be collected in-store, as well.
- Personalized information. The same data used to analyze demographics can be used to curate a highly specific sales experience for each person.
- Timed interactions. When were certain customers in the store? When were they browsing online, and how long did they take to make a purchase?
Without real-time capabilities, all of this data can be used to a limited extent. Retailers can track patterns over time, target demographics, improve products, and even send personalized offers to specific customers.
But this isn’t enough. To be effective, retailers need a unified customer data platform that is reactive to a single customer’s activities across channels. One survey found that 60% of companies rated their ability to leverage real-time data as “extremely important.”
Why is this? Consumers have grown to expect marketing and advertising to be not only personalized, but also timely. They like to be served suggestions that are relevant to their current needs, particularly while they’re in “shopping mode.” When marketing is irrelevant or served at the wrong time, we find it grating and irritating. Effective real-time marketing and advertising should feel natural for the customer.
Say a customer visits an online retailer. Their data is collected and analyzed, and the system identifies them. As they click, they’re served recommendations tailored not just to their current session, but to their past purchases and other previously collected data. If they leave the site without a purchase, they receive a coupon by email later that night. This requires a highly reactive, real-time pipeline.
Apart from personalization, real-time data helps in the following areas:
- Conversion tracking. Determining how ads or marketing techniques lead to sales.
- Real-time segmentation. Segmenting customers actively during their journey.
- Budgeting. Keeping track of ad spending and automatically staying within the defined budget.
4. Inventory management
Along with marketing, inventory management is another important use-case for real-time analytics in the commercial sphere.
Take the classic example of an online store. A finite amount of each item is known to be in stock. The system takes into account each purchase, as well as items that are left in abandoned shopping carts. This accomplishes two main tasks:
- Prevents conflict, for example, erroneously selling more items than are available.
- Tracks supply, helping managers plan orders and stocking.
Inventory management has a range of other applications, such as warehouse management, and ticket sales for airline flights, shows, and events.
Inventory management is a delicate balance of supply and demand. Surplus and shortage both have financial penalties. Real-time data allows analysts to make forward-thinking inventory decisions rather than simply reacting. Emerging patterns can be detected right away, which helps prevent losses.
For instance, a significant rise and fall in demand might tell an airline to add more flights to a specific route, or cancel them. They can then plan for the aircraft and crew considerations well in advance as they make more tickets available. Similarly, analytics can tell a warehouse manager when they should back-order a specific item, whether it makes sense to buy in bulk, or if they should cancel shipments.
5. KPIs, OKRs, and business intelligence in general
Real-time analytics can tell you how your business — any business — is performing day to day and minute to minute.
This example may seem vague, but that’s because it’s so universally applicable. Every business should be measuring performance at least on a basic level. The buzz around business intelligence (transforming data into insights that inform business decisions) has taken off in recent years as data tools have become more accessible for organizations of all sizes.
Key performance indicators (KPIs) and objectives and key results (OKRs) are a pretty basic concept in this arena. But defining the right KPIs and OKRs, and using them effectively can be more challenging than we anticipate. Chasing metrics can result in a disconnect between our vision and what’s actually happening in the real world.
When we make business intelligence adaptive and reactive in real-time, a couple of things happen that can help alleviate this disconnect.
- It’s easier to transform metrics into usable daily tools, instead of something for the executive suite’s quarterly slide deck.
- Mechanical, time-consuming tasks like running daily reports are eliminated. Workers can focus on using data as a helpful tool.
- Insights are generated quicker, so you can both leverage insights faster and proactively detect when your strategy might need to change.
Of course, real-time metrics must be used responsibly to be helpful. It’s important to evaluate which metrics are actually valuable in real time, and which should be reserved for long-term analytics. This should be part of your team’s strategic planning.
Even if you don’t need to run data as fast as, say, a financial institution needs to detect fraud, a flexible data pipeline that integrates data at all time scales is a valuable tool. This way, you can use a single source of truth to, for example, update your sales team’s mobile dashboards and generate quarterly reports.
How you can leverage real-time data
Real-time data analysis powers many of the insights we rely on as business managers and take for granted as consumers. Its use-cases range beyond those discussed here: stock trading, cyber security, environmental regulation, cargo, gaming, and more.
So, how can you use real-time data more effectively in your organization?
Implementing a robust, user-friendly, real-time data pipeline can be a daunting task. This is especially true if you don’t want to overhaul your existing architecture or are unsure how, exactly, to tackle your unique use-case.
At Estuary, that’s our specialty. We help you build and maintain affordable pipelines that power real-time data analytics without unnecessary friction.
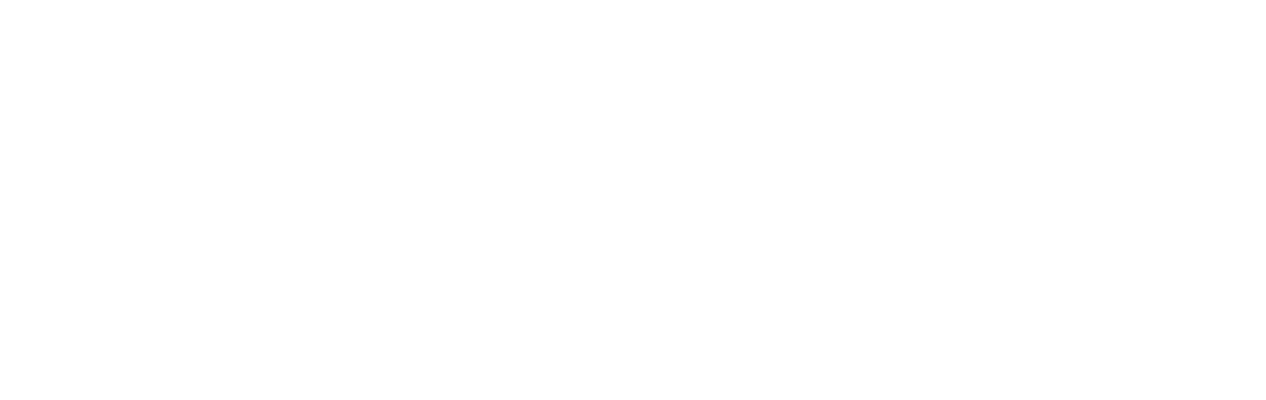
Author
Popular Articles
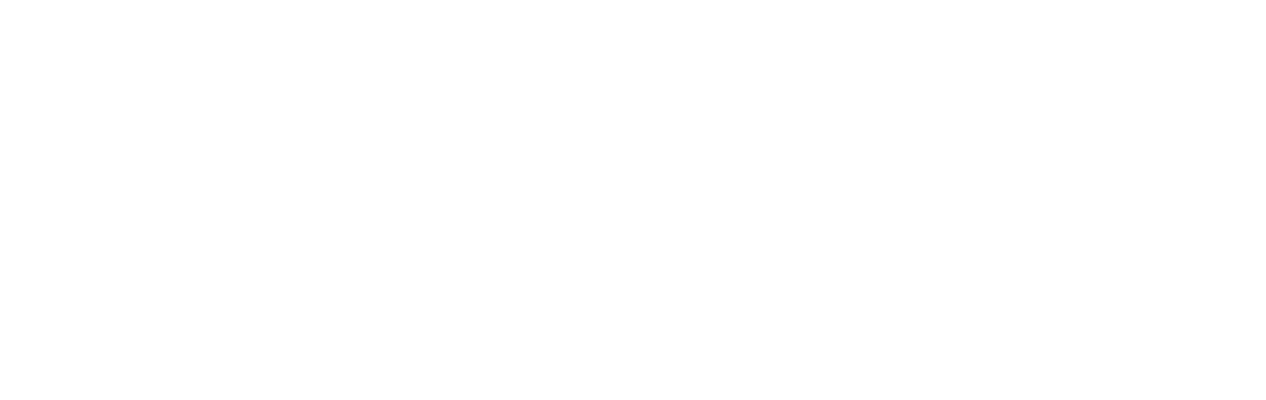